Labeling Tools for Machine Learning: Enhancing Your Data Annotation Processes
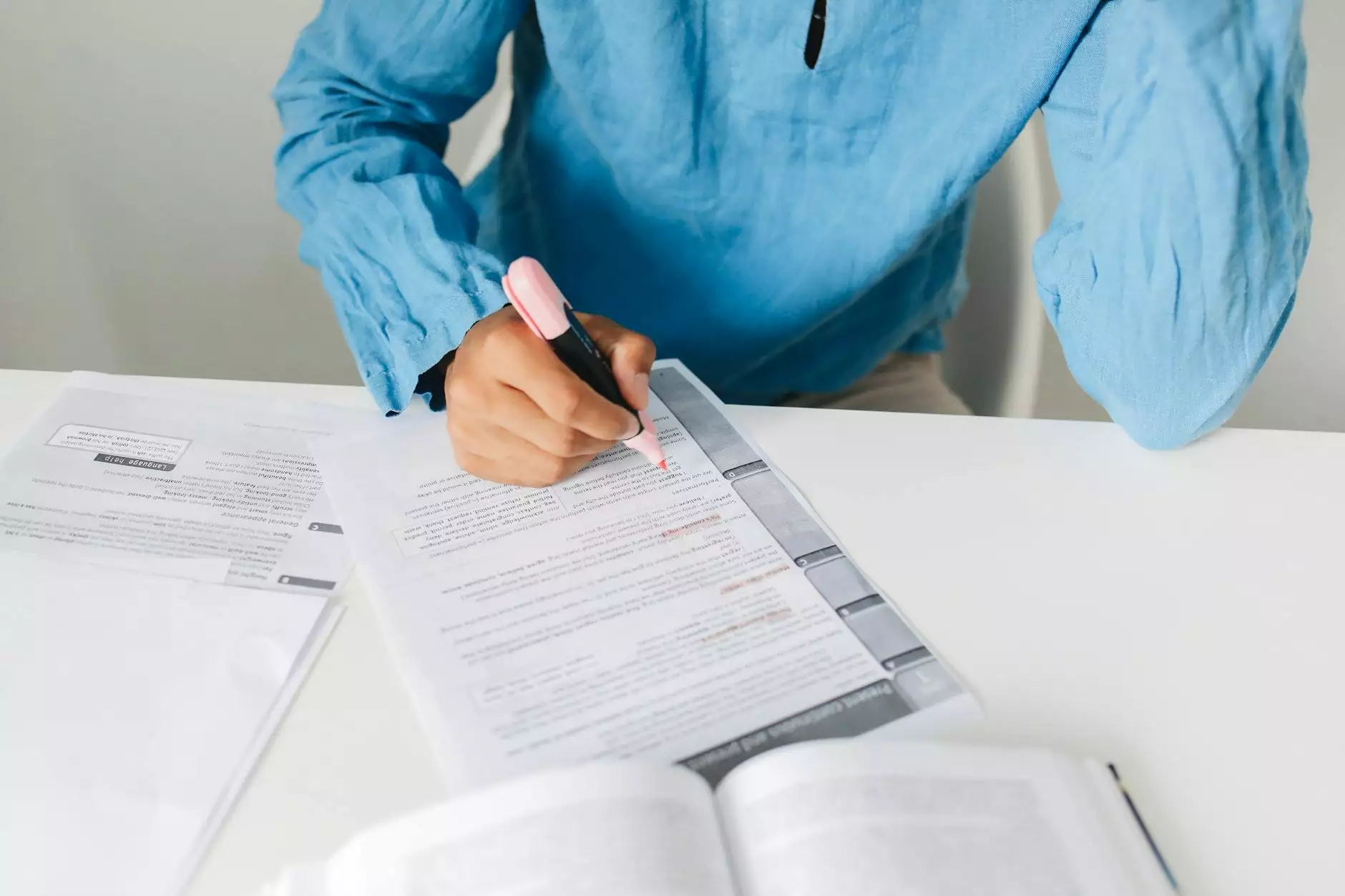
In today’s data-driven world, machine learning and artificial intelligence have become foundational technologies that drive innovation across various industries. However, one of the significant challenges in leveraging these technologies is the need for high-quality, labeled data. This is where labeling tools for machine learning come in, offering businesses powerful solutions that streamline the data annotation process.
Understanding the Importance of Data Labeling
Data labeling is the process of annotating data samples with meaningful tags that machine learning algorithms can understand. Whether it’s images, text, or audio, labeled data serves as the training ground for models, influencing the accuracy and effectiveness of predictions made by AI systems. Without proper labeling, even the most sophisticated algorithms can produce inaccurate results, leading to poor business decisions.
The Role of Labeling Tools
Labeling tools are software applications designed to assist users in efficiently annotating data. These tools provide a user-friendly interface and a set of features that facilitate the creation of labeled datasets. By using appropriate labeling tools for machine learning, businesses can:
- Reduce Annotation Time: Automating or simplifying the annotation process helps in speeding up the data preparation stage.
- Enhance Consistency and Quality: Many tools include features that enforce consistent labeling practices, reducing human errors.
- Manage Large Datasets: Scalable tools make it easy to handle vast amounts of data, enabling businesses to train their models effectively.
- Collaborate Efficiently: Some tools support team collaboration features, allowing multiple annotators to work together seamlessly.
Popular Labeling Tools for Machine Learning
Choosing the right labeling tool is crucial for optimizing your data annotation workflow. Here are some of the most popular labeling tools that businesses across various sectors utilize:
1. Labelbox
Labelbox is a powerful, cloud-based tool that provides an intuitive interface for annotating images and videos. With collaborative features and integration capabilities, Labelbox allows teams to label data efficiently while maintaining high-quality standards.
2. Amazon SageMaker Ground Truth
This service from Amazon Web Services offers a comprehensive labeling solution that combines automated data labeling with human verification. It’s particularly advantageous for businesses already utilizing AWS for their machine learning needs.
3. Supervisely
Supervisely is a versatile platform that caters to various labeling needs, including image segmentation and 3D point cloud annotation. Its robust features enable users to build and manage datasets effectively.
4. CVAT (Computer Vision Annotation Tool)
CVAT is an open-source annotation tool developed by Intel. It’s designed for both image and video annotation and is suitable for teams looking for a customizable solution.
5. Prodigy
Prodigy focuses on text and image annotation with a unique workflow that leverages active learning. This allows users to prioritize the most informative examples for labeling, ultimately increasing the efficiency of the annotation process.
Factors to Consider When Choosing Labeling Tools
When selecting labeling tools for machine learning, consider the following factors:
- Type of Data: The tool should support the specific type of data you are working with, whether it be images, text, audio, or video.
- Usability: An intuitive interface is crucial for ensuring that your team can work efficiently without extensive training.
- Collaboration Features: Look for tools that facilitate teamwork and communication among annotators.
- Integration Capabilities: Ensure that the labeling tool can integrate with your existing workflows and technology stack.
- Cost: Analyze your budget and evaluate the pricing structure of the tool to ensure it provides value for your business.
Implementing Labeling Tools in Your Workflow
Once you have selected the appropriate labeling tools for machine learning, the next step is to implement them into your workflow. Here’s a step-by-step guide on how to do this effectively:
Step 1: Define Your Goals
Before you start the labeling process, outline your objectives. Determine what kind of data you need, the criteria for labeling, and how the annotated data will be used in your machine learning models.
Step 2: Choose the Right Tool
Based on your requirements, select a labeling tool that aligns with your goals. Take advantage of trial periods or demos to evaluate the tool’s usability.
Step 3: Train Your Team
Provide adequate training to your team members on using the chosen labeling tool. This can prevent errors and misunderstandings during the annotation process.
Step 4: Start Annotating
Begin the data annotation process. Monitor the progress and quality of the labeling to ensure that the data meets the necessary standards.
Step 5: Review and Validate
Once the data is labeled, conduct thorough reviews to validate the annotations. Involve multiple reviewers to minimize bias and improve quality assurance.
Future Trends in Data Labeling
The future of data labeling is poised for transformation as advancements in technology continue to evolve. Here are some trends to watch:
- Automated Labeling Solutions: As AI algorithms improve, so will automated labeling capabilities. Tools leveraging machine learning for pre-labeling are expected to become more common.
- Active Learning: Active learning techniques will allow models to request specific labels for data points they are uncertain about, optimizing the annotation process.
- Crowdsourcing Labeling: Expect to see more platforms that connect businesses with teams of annotators from around the world, bringing diversity and scalability to data labeling.
- Enhanced Collaboration Tools: Tools that facilitate real-time collaboration will become essential as remote work becomes more prevalent.
Conclusion
In conclusion, the success of machine learning applications heavily depends on the availability of high-quality labeled data. By investing in effective labeling tools for machine learning, businesses can streamline their data annotation processes, improve accuracy, and ultimately enhance their decision-making capabilities. As the technology continues to advance, staying updated with the latest trends and tools in data labeling will ensure that businesses maintain a competitive edge in the ever-evolving landscape of AI technology.
With the right approach to data labeling, organizations can unlock new potentials, drive innovation, and create significant impacts in their respective industries.